
In: Proceedings of the IEEE/CVF Conference on Computer Vision and Pattern Recognition, pp. Liu, L., Jia, X., Liu, J., Tian, Q.: Joint demosaicing and denoising with self guidance. In: 2019 IEEE International Conference on Image Processing (ICIP), pp. Koskinen, S., Yang, D., Kämäräinen, J.K.: Reverse imaging pipeline for raw RGB image augmentation. Kingma, D.P., Ba, J.: Adam: a method for stochastic optimization. Jin, Q., Facciolo, G., Morel, J.M.: A review of an old dilemma: demosaicking first, or denoising first? In: Proceedings of the IEEE/CVF Conference on Computer Vision and Pattern Recognition Workshops, pp. Guo, S., Liang, Z., Zhang, L.: Joint denoising and demosaicking with green channel prior for real-world burst images. In: Proceedings of the IEEE/CVF International Conference on Computer Vision, pp. 1251–1258 (2017)Įhret, T., Davy, A., Arias, P., Facciolo, G.: Joint demosaicking and denoising by fine-tuning of bursts of raw images. In: Proceedings of the IEEE Conference on Computer Vision and Pattern Recognition, pp. 3291–3300 (2018)Ĭhollet, F.: Xception: deep learning with depthwise separable convolutions.
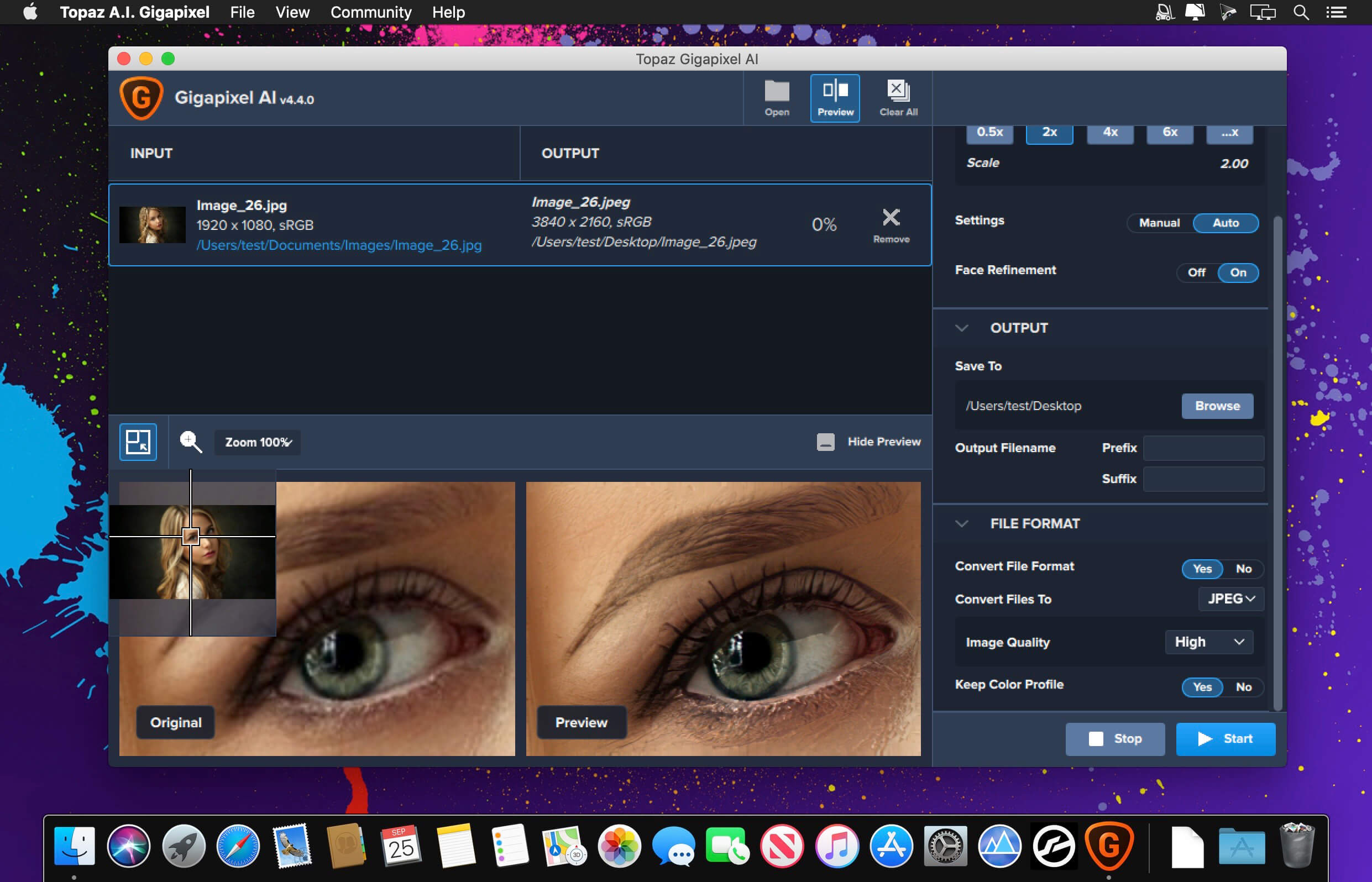
16(8), 2080–2095 (2007)īrooks, T., Mildenhall, B., Xue, T., Chen, J., Sharlet, D., Barron, J.T.: Unprocessing images for learned raw denoising. Image denoising by sparse 3-D transform-domain collaborative filtering. Experimental results on SID and ELD dataset demonstrate our proposed SUnet++ outperform the state-of-the-arts methods in term of subjective and objective results, which further validates the robust generalization of our proposed method. We introduce Unet++ to adapt the model to other datasets, and then replace the conventional convolutions of Unet++ with M sets of depthwise separable convolutions, which greatly reduced the number of parameters without losing performance.
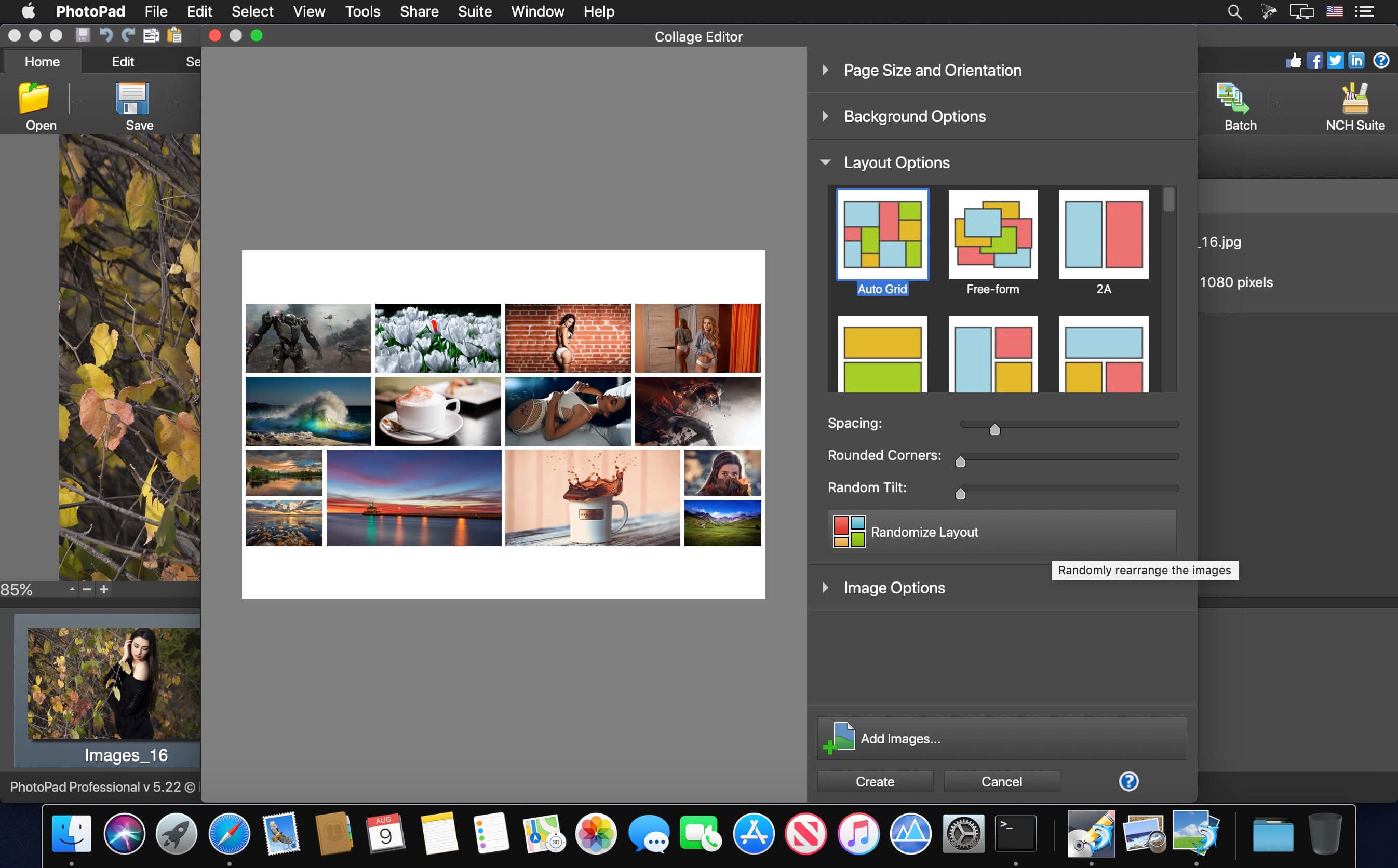
In order to address this problem, we present a separable Unet++ (SUnet++) network structure to improve the generalization ability of the joint denoising and demosaicing method for extreme low-light images. Existing methods use neural networks to automatically learn the mapping from extreme low-light noise RAW images to long-exposure RGB images for jointly denoising and demosaicing of extreme low-light images, but the performance on other datasets is unpleasant. Despite the rapid development of photography equipment, shooting high-definition RAW images in extreme low-light environments has always been a difficult problem to solve.
